The acceleration of Artificial Intelligence (AI) technology adoption across industries has indicated widespread organisational acceptance of the novel technology. The advent of this new technology has brought more efficient and creative possibilities. However, the use of language models in AI raises concerns about trustworthiness in its training and the output it generates. This leads us to the question: How can we leverage existing technologies to bridge this lack of trust?
This article explores the challenges surrounding trust in AI system inputs and outputs, how blockchain could be the solution to establish trust, and various integration challenges that need to be accounted for.
Trust challenges of AI systems
Since the launch of ChatGPT in November of 2022, organisations have made enormous strides to implement AI within their technological systems. Enthusiasm for this new technology promising productivity enhancement, has also generated discussions on whether we can trust these systems. Who has been training these AI models? How can we be sure that the information output it generates is reliable and unbiased?
Transparency of AI language models
AI systems require a large language model (LLM) to learn and have a basis to create outputs from. However, due to the lack of transparency surrounding what goes into the LLMs, doubts arise on data input authenticity and reliability.
Authenticity: If the origin, quality, and representativeness of the input data are not transparent, it raises concerns about the authenticity and reliability of the model’s understanding.
Reliability: Lack of clarity regarding the source of training data leads to scepticism about the model’s ability to accurately reflect real-world scenarios and diverse perspectives. This creates uncertainty on whether the model’s output can be trusted and used accordingly.
Explainability: There is a lack of self-explanation in AI systems on how a specific outcome is generated when prompted. This poses a significant hurdle to understanding and trusting AI’s outputs. What data did the system pool together to carry out its decision-making process when prompted?
Ethical standards in AI training
AI systems often make decisions based on complex algorithms and data that include principles governing the training of AI models. Thus creating the need for a standardised set of ethical guidelines governing the training of AI models.
One primary concern is the ethical framework guiding the selection of data used in training these systems. The opaqueness surrounding the training data raises ethical concerns, particularly related to bias. If the data used to train AI models contain biases, the model may unintentionally perpetuate those biases in its outputs. Without transparency, it becomes challenging to identify and address biases in the training data. Therefore making it difficult to avoid potential negative consequences.
Authenticating human vs. AI output
Another significant challenge revolves around the ability to distinguish between outputs generated by AI systems and those by human intelligence. Can we reliably identify whether the output presented is a result of human thought or an algorithmic process?
Authentication is particularly crucial in scenarios where information transparency is essential, such as journalism, content creation, or academia. This distinction between human and AI-generated content also carries ethical implications. It prompts discussions on attribution, accountability, and the potential influence these systems might have in shaping public opinion. As AI becomes more integrated into society, different mechanisms are needed to transparently demonstrate the origin of information.
Output challenges
When speaking of trust within AI, we must also evaluate the ownership and intellectual property rights of AI-generated output. The challenge lies in determining whether outputs are established through AI’s novel agency or the accumulation of various other sources that warrant intellectual property rights.
The artwork industry can be used to illustrate the debate on whether generative AI should give credit to artists. Since the system draws inspiration from its training model, would the new artwork’s ownership belong to AI? This debate highlights legal and ethical dilemmas, due to a lack of established frameworks that promote fair compensation for contributors. As AI systems evolve, there is a pressing need to redefine traditional notions of ownership. Acknowledgment of the collaborative nature of AI development is needed while safeguarding the rights of those involved in the generative process.
Role of blockchain in establishing trust
Based on the trust challenges discussed above, blockchain technology could be the solution as a trust layer for AI. Blockchain’s decentralised design, using transparent and immutable data records, guarantees universal accessibility, enhanced authentication, and verification processes for all users.
Ensuring transparency in data processes
One of the fundamental challenges in trusting AI, as discussed, lies in the lack of transparency regarding data input processes. Blockchain can resolve the transparency issue by recording every step of the AI training and data input process. This ensures that the origin and reliability of the data used to train AI models are traceable and verifiable to reflect real-world conditions.
By leveraging blockchain, organisations facilitating AI technology can instil confidence in stakeholders regarding the reliability of its AI-generated outputs. This safeguards against potential misuse and establishes a foundation for accountability in the development and deployment of AI.
Tracing decision-making processes
Transparency enabled by blockchain technology can address the inherent explainability challenges associated with AI’s black-box nature. Establishing a transparent trail of the data and model processes integrated enhances the reliability of AI decisions.
The availability of a comprehensible trail can address the lack of confidence and scepticism present in generative AI. Traceability encourages independent evaluation, empowering users to revisit and assess AI’s decision-making steps, thereby contributing to a more accountable and trustworthy AI environment.
Leveraging immutability to validate data input reliability
The immutable nature of blockchain technology contributes to providing security to data records and data transactions. Every piece of information entered into the blockchain is time-stamped and linked to previous data blocks, creating an unbroken chain of custody. This not only enhances the accountability of data sources but also enables stakeholders to verify the authenticity of inputs. As a result, users can confidently rely on the integrity of the data feeding into AI algorithms, knowing that any attempt to manipulate or compromise the information would be immediately detectable. On the other hand, blockchain can be used to drive sustainability goals for successful operations in the long run. For further understanding of how blockchain can sustain long-term ESG goals for organisations, refer to this ebook on using blockchain for sustainability.
Authenticating human vs. machine contributions
Distinguishing between outputs generated by human intelligence and those crafted by AI is critical. The widespread use of AI in optimising many of our daily processes is bound to be used in other various domains, such as work-related situations and advisory roles. This has caused increasing evaluation on whether information distributed is completely human or contains traces of AI contribution.
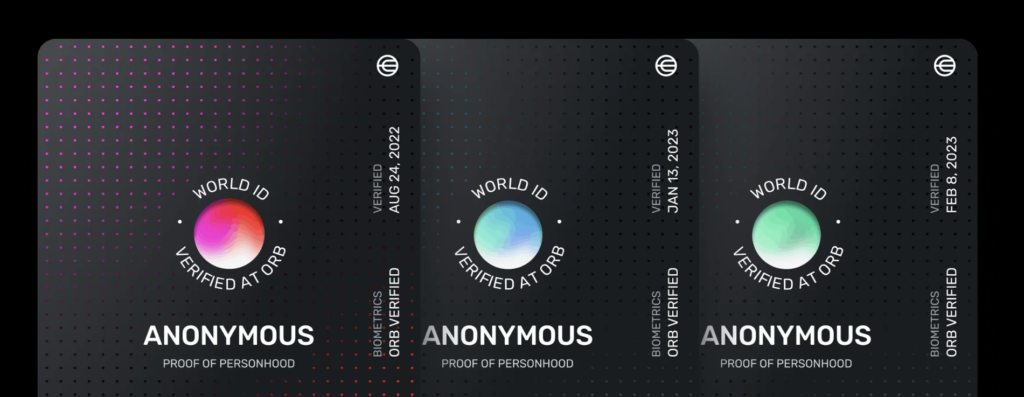
Thus the use of smart contracts in the blockchain ecosystem provides a mechanism for creating verifiable identities for both human and machine contributors. OpenAI’s WorldID initiative is an example of how smart contracts can be employed to authenticate the origin of contributions. Its application will enable a clear distinction between human and AI-generated outputs. Their initiative could address future concerns about accountability and ethical considerations in various domains such as authentic journalism and recognition.
Establishing auditable trails for tracing errors
In the complex landscape of AI, errors and biases in outputs can have significant consequences for end users. Blockchain-based systems can offer the solution of an auditable trail that records every transaction and interaction within the AI ecosystem. In the event of errors or biases in AI outputs, this auditable trail provides a comprehensive analysis of the training data and its parameters, facilitating the identification and rectification of issues. This auditable trail also serves as a critical tool for post hoc analysis, allowing for continuous improvement and refinement of AI algorithms to meet evolving ethical and performance standards.
Challenges between AI and blockchain integration
The integration of AI and blockchain technology may provide us with a starting point in establishing trust, however, there are still potential challenges that experts should address when considering this integration.
Scalability
One prominent concern is the scale and scope of AI applications. AI systems often work with massive datasets, and to fully integrate them with blockchain, a highly efficient, low-cost network is essential. However, the transactional and resource costs associated with blockchain networks remain a significant hurdle.
Cost efficiency
Initial setup costs can involve acquiring the necessary hardware, software, and infrastructure to support both AI algorithms and blockchain networks. Maintenance costs also arise as ongoing updates and improvements are essential to keep both technologies optimised and secure. Transaction costs associated with blockchain networks, such as fees for data storage and processing, can accumulate. Particularly when dealing with large-scale AI applications that require frequent interactions with the blockchain. Lastly, organisations will need to invest in skilled professionals who can navigate the complexities of integrating AI and blockchain, adding to the overall human resource expenses.
Interoperability
There are various blockchain platforms available, and achieving interoperability between them poses a challenge since smart contracts and AI applications might need to interact across different blockchain networks. AI models and algorithms may be developed using different frameworks and technologies. Therefore, ensuring a seamless integration with blockchain platforms can be a complicated process.
Regulatory Compliance
The topic of interoperability across both technologies also highlights regulatory challenges on two fronts. The decentralised and global nature of blockchain introduces uncertainty, as compliance requirements vary across jurisdictions. Simultaneously, the use of AI, particularly in sensitive domains, is subject to rigorous regulatory scrutiny with a focus on data privacy, transparency, and accountability. Combining these technologies requires a dual compliance effort of addressing regulations specific to each domain and staying up to date with technical developments, to develop a standardised process for compliance.
Conclusion
The widespread integration of AI technology has prompted critical discussions surrounding transparency, authentication and ethical standards in AI language models. Blockchain, through its transparent and immutable nature, emerges as a promising solution to address these concerns. It offers transparency in data processes, traceability of decision-making, and validation of data input reliability. However, the integration of AI and blockchain presents a set of challenges, such as scalability, interoperability, regulatory compliance, and cost efficiency. Thus, when leveraging this integration, a balance is needed between innovation, and ethical considerations. AI developers should ensure that the collaborative development of AI is transparent, accountable, and trustworthy for the benefit of its users.